Study Finds Peripheral Blood Biomarkers Might Improve Accuracy In Diagnosing Idiopathic Pulmonary Fibrosis (IPF)
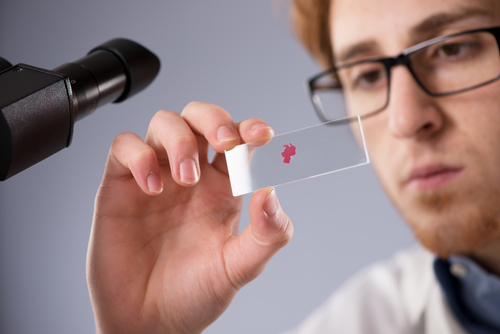
A research article published this month in the journal BMC Genomics finds that peripheral blood biomarkers might improve accuracy in diagnosing idiopathic pulmonary fibrosis (IPF) — which the National Heart, Lung, and Blood Institute describes as a disease in which tissue deep in the lungs becomes thick and stiff, or scarred, over time. IPF is a serious disease that usually affects middle-aged and older adults. There is no cure, and many people live only about 3 to 5 years after diagnosis.
The NHLBI notes that research has helped doctors learn more about IPF. As a result, they can more quickly diagnose the disease now than in the past. Also, researchers are studying several medicines that may slow the progress of IPF. These efforts may improve the lifespan and quality of life for people who have the disease.
The Open Access paper, entitled “Familial and sporadic idiopathic pulmonary fibrosis: making the diagnosis from peripheral blood” (BMC Genomics 2014, 15:902 doi:10.1186/1471-2164-15-902) is coauthored by Eric B Meltzer and Allison Ashley of Duke University Medical CenterDepartment of Medicine, Durham, NC; William T. Barry of the Department of Biostatistics and Computational Biology, Dana-Farber Cancer Institute, Boston, MA; Ivana V. Yang, Marvin I. Schwarz, and David A. Schwartz of the Department of Medicine, Anshutz Medical Campus, University of Colorado, Aurora, CO; Kevin K. Brown of the National Jewish Health, Denver, CO; Hamish Patel of Edward Via College of Osteopathic Medicine, Spartanburg, SC; Paul W. Noble of the Department of Medicine, Cedars-Sinai, Los Angeles, CA; and Mark P. Steele (Corresponding author) of the Division of Allergy, Pulmonary, and Critical Care, Vanderbilt University Medical Center, Nashville, TN.
The researchers report that gene expression profiles were obtained from 89 patients with IPF and 26 normal controls.
Samples were stratified according to severity of disease based on pulmonary function. The stratified dataset was split into subsets; two-thirds of the samples were selected to comprise the training set, while one-third was reserved for the validation set. Bayesian probit regression was used on the training set to develop a gene expression model (“Bayesian linear regression is an approach to linear regression in which the statistical analysis is undertaken within the context of Bayesian inference. When the regression model has errors that have a normal distribution, and if a particular form of prior distribution is assumed, explicit results are available for the posterior probability distributions of the model’s parameters” – Wikipedia) for IPF versus normal. The gene expression model was tested by using it on the validation set to perform class prediction. Unsupervised clustering failed to discriminate between samples of different severity.
Therefore, samples of all severities were included in the training and validation sets, in equal proportions. A gene signature model was developed from the training set. The model was built in an iterative fashion with the number of gene features selected to minimize the misclassification error in cross validation. The final model was based on the top 108 discriminating genes in the training set. The signature was successfully applied to the validation set, ROC area under the curve = 0.893, p < 0.0001. Using the optimal threshold (0.74) accurate class predictions were made for 77% of the test cases with sensitivity = 0.70, specificity = 1.00.
The coauthors summarize that by using Bayesian probit regression to develop a model, we show that it is entirely possible to make a diagnosis of IPF from the peripheral blood with gene signatures.
[adrotate group=”3″]
Results
The stratified dataset was split into subsets; two-thirds of the samples were selected to comprise the training set, while one-third was reserved for the validation set. Bayesian probit regression was used on the training set to develop a gene expression model for IPF versus normal.
The gene expression model was tested by using it on the validation set to perform class prediction. Unsupervised clustering failed to discriminate between samples of different severity.
Therefore, samples of all severities were included in the training and validation sets, in equal proportions. A gene signature model was developed from the training set.
The model was built in an iterative fashion with the number of gene features selected to minimize the misclassification error in cross validation. The final model was based on the top 108 discriminating genes in the training set.
The signature was successfully applied to the validation set, ROC area under the curve = 0.893, p
Conclusions
The article coauthors conclude that by using Bayesian probit regression to develop a model, they show that it is entirely possible to make a diagnosis of IPF from the peripheral blood with gene signatures.
The article abstract is here:
https://www.biomedcentral.com/1471-2164/15/902/abstract#
Sources:
BMC Genomics
National Heart, Lung and Blood Institute
Wikipedia
Introduction To Bayesian Thinking (Blog)