Analysis of Lung Tissue Reveals Important Genetic Drivers of IPF
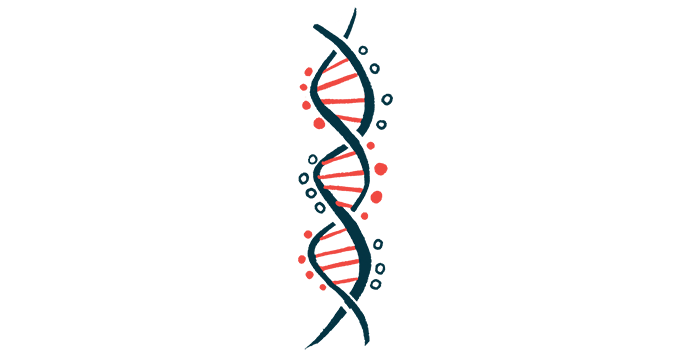
An analysis of gene activity profiles in lung tissue has identified important drivers of idiopathic pulmonary fibrosis (IPF).
The genes discovered in this integrative analysis may predict disease outcomes and be used as potential therapeutic targets for new anti-fibrotic treatments, according to researchers.
The study, “Integrative analysis of lung molecular signatures reveals key drivers of idiopathic pulmonary fibrosis,” was published in the journal BMC Pulmonary Medicine.
IPF is a chronic lung disease characterized by progressive scarring (fibrosis) of the lungs and is associated with worsening respiratory symptoms and a decline in lung function.
Disease-related profiles of gene expression, or activity, have provided insights into the development of IPF and proved helpful in predicting disease progression and responses to therapeutic interventions.
However, previous gene expression studies have involved different sized patient groups with diverse backgrounds and have used different protocols and technologies. As such, direct comparisons between these results are not possible.
A comprehensive, integrated analysis using genome-wide datasets may find disease-related gene expression profiles missing from previous studies and detect additional factors driving IPF.
Researchers at the Catholic University of Korea set out to compile gene expression data of lung tissue found in public databases to create an IPF compendium and characterize its molecular and cellular features.
The team searched databases using IPF-related terms and collected several datasets that included 585 IPF patients and 362 healthy individuals who served as controls, covering 15,447 genes in common.
They focused on genes from lung tissue that were expressed differently in those with and without IPF, dubbed differentially expressed genes (DEGs). Their aim was to look for genes with elevated gene expression (upregulated) in patients. A total of 2,967 upregulated DEGs were identified.
Network analysis found 6,658 of these genes interacted, and 1,190 genes had more than one link to other genes. Within the network, the DSP gene was identified. A variant of this gene is known to increase IPF risk. A total of 14 IPF-associated biomarkers were also in the network.
A functional analysis was then performed and two critical associated biological pathways were identified: p53 and PI3K-Akt. The PI3K-Akt pathway plays a role in the cycle, metabolism, and survival of cells, while p53 regulates the progression of the cell cycle, programmed cell death (apoptosis), and genomic stability.
Clustering analysis of DEG profiles from 585 IPF patients found two clusters that most represented the data: C1, with data from 252 IPF patients; and C2, representing 333 patients.
To distinguish disease-related features among C1 and C2, the team curated IPF-related pathways and information on cell population markers in the lung from the literature. The p53 signaling pathway was enriched in C1, whereas C2 was associated with the PI3K-Akt, TGF-beta, and FoxO signaling pathways, as well as cellular senescence — an inability to progress through the cell cycle.
Cells enriched in C1 included immune dendritic cells and cells with cilia — hair-like structures that extend from the cell surface. C2 was associated with cells that line the tiny air sacs in the lungs (alveoli), immune macrophages, and fibroblasts — the most common type of connective tissue cell, which is overactive in IPF. There was no difference in lung function between the two groups.
A correlation assessment between enriched pathways and lung function parameters found p53 and the pro-inflammatory interleukin-17 pathways most strongly correlated with worse lung function. These pathways were also associated with increased cellular senescence and apoptosis.
“This underscores a close relationship between the p53 signaling pathway, [alveolar] senescence, and progressive disease in IPF, suggesting the need for a stratified approach to patient management based on the molecular signature of lung tissue,” the researchers wrote.
IPF biomarkers can improve disease classification and help with diagnosis, therapeutics, and outcomes (prognosis). In total, the researchers found 38 molecular biomarkers in the IPF gene expression profiles. Of these, 15 were categorized into risk variants, 10 were diagnostic markers, and 18 were prognostic markers.
Gene expression levels in 30 biomarkers were significantly different between C1 and C2, with 11 genes more highly expressed in C1 over C2, including the DSP gene (2.2-times higher expression).
The MUC16 gene, which encodes for a cell surface protein essential in forming a protective mucous barrier, was 3.65-times higher. MUC16 is also a strong predictor of disease progression and mortality in IPF. In addition, the prognostic scores of subgroup C1 were significantly higher than those in C2.
An analysis to prioritize genes that may cause IPF found 119 key driver genes, of which 30 were DEGs. The RPS6 gene was the top-ranked gene, followed by RPS6KA1, VCAM1, ITGA4, and CSNK1A1. Additionally, FN1, ICAM1, CDK2, CDK1, CDKN2A, and CKDN1A were also ranked as key driver genes, all of which “may serve as promising targets for therapeutic intervention,” the team wrote.
Finally, the researchers collected information on the therapeutic targets of 11 current approved treatments and two investigational therapies, and calculated and compared scores related to their molecular targets. Compared to other medications, N-acetylcysteine, imatinib, and Ofev (nintedanib) had the highest scores and were “assumed to be more effective owing to their multiple targets,” the team added.
“An integrative molecular analysis of IPF lungs identified two molecular subgroups with distinct pathobiological [disease-related] characteristics and clinical prognostic scores,” the researchers wrote. “This approach provided molecular insights to support the prediction of clinical outcomes and the selection of therapeutic targets in IPF patients.”